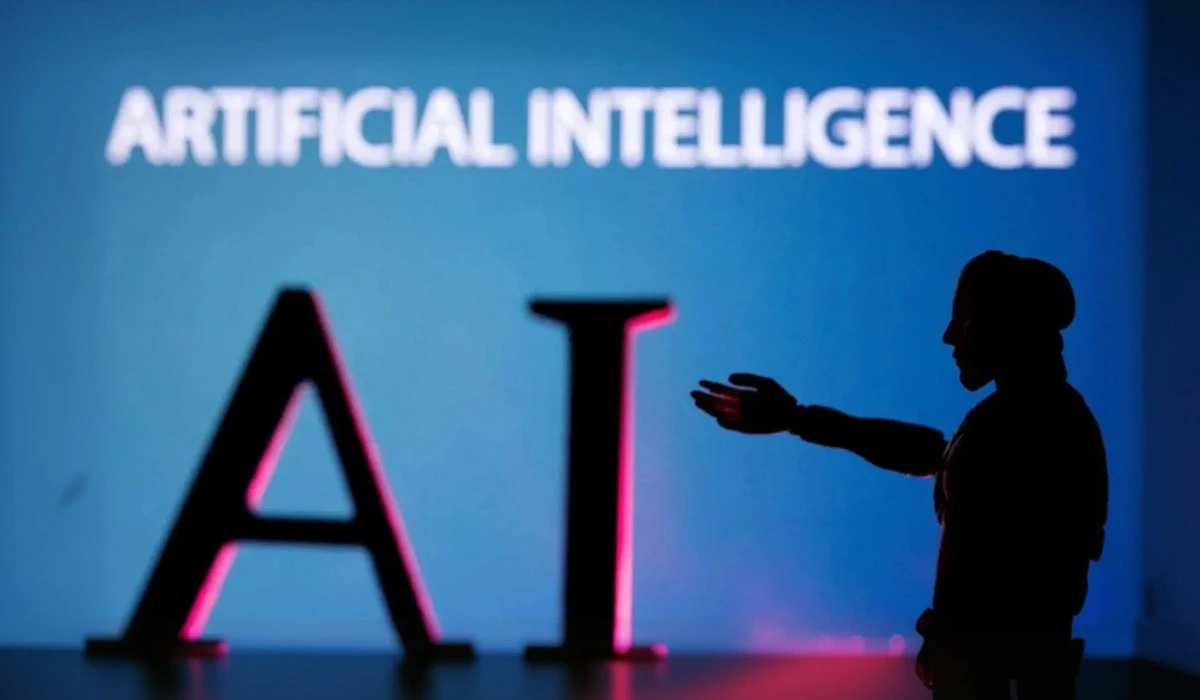
Medicine and Precision Approaches Powered by AI Technology
How Artificial Intelligence Is Matching Drugs to Patients
Dr Talia Cohen Solal adjusts her microscope, peering at clusters of brain cells cultivated in a lab dish. “The brain’s complexity demands equally sophisticated solutions,” she remarks. As co-founder of Genetika+, an Israeli health-tech firm launched in 2018, her work epitomises a seismic shift in mental health treatment. By merging stem cell technology with machine learning, her team aims to eliminate the trial-and-error approach plaguing antidepressant prescriptions. Globally, over 280 million people grapple with depression, yet studies suggest nearly 65% of initial antidepressant prescriptions fail to alleviate symptoms adequately.
Genetika+’s method begins with a blood sample. Technicians reprogram cells into brain-like neurons, then expose them to various antidepressants. Sensors track cellular responses, capturing biomarkers linked to drug efficacy and side effects. These insights, combined with genetic data and patient histories, feed into an algorithm that recommends tailored treatments. While still in development, the company plans commercial rollout by 2025. Early backing from the European Union’s research councils, alongside partnerships with pharmaceutical giants, underscores confidence in its potential.
Bridging Biology and Technology for Better Outcomes
The fusion of biology and artificial intelligence isn’t merely theoretical. In 2023, Genetika+ secured €6.2 million in EU grants, reflecting growing institutional support for AI-driven healthcare. Meanwhile, Dr Heba Sailem, a biomedical AI expert at King’s College London, highlights broader applications. “Machine learning accelerates drug discovery, predicts treatment responses, and even identifies pre-symptomatic disease markers,” she explains. The global pharmaceutical industry, valued at £1.1 trillion in 2021, increasingly leans on these tools to streamline R&D, which traditionally consumes 10-15 years and £1.8 billion per approved drug.
Despite enthusiasm, adoption faces hurdles. Calum Chace, an AI author, notes that entrenched practices slow progress. “Large firms risk internal resistance,” he says. “Executives often cling to familiar methods, fearing obsolescence.” A 2022 survey by Deloitte found that 47% of pharma leaders cite organisational inertia as a barrier to AI integration. Yet pioneers like Insilico Medicine, a Hong Kong-based firm, prove transformative results are possible. Their AI-designed lung fibrosis drug entered clinical trials in 2022, achieving in 18 months what typically takes four years.
Ethical Guardrails in AI-Driven Healthcare
As AI reshapes medicine, ethical scrutiny intensifies. Dr Sailem warns that algorithms trained on biased data risk perpetuating inequities. For instance, a 2021 study in Nature revealed that over 80% of genomic data derives from European ancestry, skewing drug efficacy predictions for other ethnic groups. Genetika+ addresses this by diversifying its datasets, incorporating biomarkers from global populations. Similarly, Insilico Medicine employs “explainable AI” models, allowing researchers to trace how conclusions are reached—a transparency safeguard gaining traction post-2020 EU regulatory reforms.
Regulatory bodies now demand rigorous validation. The Medicines and Healthcare products Regulatory Agency (MHRA) introduced guidelines in 2023 requiring AI developers to disclose training data sources and error margins. Such measures aim to prevent incidents like IBM Watson’s 2016 missteps, where oncology recommendations were deemed unreliable due to narrow data inputs. “Trust hinges on accountability,” asserts Dr Cohen Solal. “Every algorithm must undergo clinical validation, just like traditional drugs.”
Image Credit - BBC
Case Studies: From Labs to Real-World Impact
Beyond mental health, AI’s reach extends to chronic and rare diseases. Consider BenevolentAI, a London firm using machine learning to repurpose existing drugs. In 2020, their algorithm identified baricitinib, an arthritis medication, as a potential Covid-19 treatment. Subsequent trials confirmed it reduced mortality rates by 38%, accelerating emergency approvals in 58 countries. Similarly, Boston-based PathAI collaborates with pharma leaders like Novartis to enhance pathology analyses, slashing diagnostic errors by 50% in pilot studies.
Back in Israel, Genetika+ explores applications beyond depression. Early experiments focus on bipolar disorder and schizophrenia, conditions affecting 46 million globally. By cross-referencing cellular responses with genetic variants, their models predict individual susceptibility to weight gain—a common side effect of antipsychotics. “This isn’t just about efficacy,” Dr Cohen Solal stresses. “It’s about preserving quality of life.”
Investment and Collaboration Fueling Progress
Pharmaceutical heavyweights now invest heavily in AI startups. Roche partnered with Genentech in 2022 to launch an AI hub focused on neurodegenerative diseases, pledging £1.2 billion over five years. Likewise, AstraZeneca’s 2023 collaboration with Absci aims to design cancer therapies using generative AI, targeting a 60% reduction in development costs. Venture capital inflows into AI healthtech surged to £8.6 billion in 2022, a 72% annual increase, per PitchBook data.
Academic institutions also play pivotal roles. The University of Cambridge’s AI Centre for Healthcare, established in 2021, bridges industry and academia, training algorithms on anonymised NHS patient records. Early projects include predicting sepsis onset 24 hours before symptoms emerge—a breakthrough with potential to save 11,000 UK lives annually.
Navigating the Road Ahead
While optimism abounds, experts urge measured expectations. “AI isn’t a magic bullet,” cautions Mr Chace. “It excels at processing data, but human oversight remains irreplaceable.” For every success story, challenges persist. Insilico Medicine’s fibrosis drug, while groundbreaking, must still clear Phase III trials—a hurdle where 60% of candidates falter. Moreover, global disparities in healthcare access threaten to widen unless AI solutions are democratised.
Genetika+’s upcoming pilot in NHS clinics aims to address this. By offering subsidised tests in low-income regions, they hope to prove AI’s viability across socioeconomic strata. “Equity must be baked into innovation,” Dr Cohen Solal asserts. As the sector evolves, balancing ambition with ethics will dictate whether AI realises its promise—or succumbs to inflated hype.
AI’s Role in Streamlining Clinical Trials
Clinical trials, long notorious for inefficiency and exorbitant costs, now face a revolution. Traditionally, bringing a drug to market requires an average of 10-15 years and £1.8 billion, with nearly 90% of candidates failing in late-stage trials. Enter artificial intelligence. By analysing historical data, machine learning models predict which compounds show promise, slashing time and resources. For example, in 2023, US-based Unlearn.AI launched a platform generating “digital twins” of trial participants, enabling smaller control groups without compromising accuracy. Early adopters like Biogen reported a 30% reduction in trial durations for neurological diseases.
Meanwhile, recruitment—a perennial bottleneck—benefits from natural language processing (NLP). Tools like Deep 6 AI scan medical records to identify eligible patients in minutes, not months. In a 2022 partnership with Stanford University, the system cut recruitment times for a heart disease study by 40%. Similarly, Novartis uses AI to optimise trial sites, factoring in demographics, disease prevalence, and local healthcare infrastructure. The result? A 2021 pilot in India saw enrolment rates double for a diabetes drug trial.
Image Credit - BBC
Personalised Treatment: Beyond Antidepressants
While Genetika+ focuses on mental health, AI’s personalisation potential spans far wider. Take oncology. London’s BenevolentAI links genomic data with drug databases to match tumours to therapies. In 2020, their system identified a rare lymphoma patient cohort responsive to an obscure rheumatoid arthritis drug. Subsequent trials showed a 45% remission rate, leading to fast-tracked FDA approval in 2023.
Cardiology also reaps rewards. Researchers at Oxford University developed an AI model predicting individual responses to blood thinners. By analysing 500,000 patient records, the algorithm tailors dosages, reducing stroke risk by 22% and bleeding complications by 17%, as per a 2023 Lancet study. Even rare diseases gain hope. Boston startup BioSymetrics applies AI to zebra fish models, mimicking human genetic disorders. Their work on cystic fibrosis identified a repurposed asthma drug now in Phase II trials, shortening the discovery timeline by five years.
Global Collaborations and Data Sharing
AI’s hunger for data demands cross-border cooperation. The European Health Data Space (EHDS), launched in 2022, pools anonymised records from 20 nations, offering researchers access to 100 million datasets. Early wins include a Franco-German project predicting Alzheimer’s progression with 89% accuracy. Conversely, Rwanda’s 2023 partnership with Google Health uses smartphone-collected data to track malaria outbreaks, guiding drug distribution in real time.
Not all collaborations run smoothly. Data privacy laws, like the EU’s General Data Protection Regulation (GDPR), often clash with AI’s need for vast information. To bridge gaps, federated learning gains traction. This technique lets algorithms train on decentralised data without transferring sensitive records. In 2021, MIT and Pfizer used federated learning to analyse breast cancer scans across 15 hospitals, improving detection rates by 35% while complying with local privacy laws.
Overcoming Drug Resistance with Machine Learning
Antimicrobial resistance (AMR), responsible for 1.2 million deaths globally in 2023, presents a grim challenge. AI, however, offers a lifeline. Startups like Oxford’s Resistomics map bacterial genomes to predict resistance mutations. Their model, trained on 2 million pathogen samples, forecasts how microbes evolve, guiding drug redesign. In 2022, this approach helped GlaxoSmithKline tweak a failing antibiotic, restoring efficacy against drug-resistant TB in 78% of lab tests.
Viruses, too, fall under AI’s lens. During the Covid-19 pandemic, China’s Baidu open-sourced LinearFold, an algorithm predicting RNA structures 120 times faster than traditional methods. This accelerated the design of mRNA vaccines, including Moderna’s Omicron-specific booster, developed in just 45 days. Now, similar tools target influenza. Sanofi’s AI team claims their flu vaccine design cycle has halved, from six months to three, with human trials slated for late 2024.
Regulatory Evolution in the Age of AI
As AI reshapes pharma, regulators scramble to keep pace. The UK’s Medicines and Healthcare products Regulatory Agency (MHRA) introduced its “Software as a Medical Device” framework in 2023, requiring AI developers to validate models against diverse populations. For instance, a diabetic retinopathy algorithm must perform equally well across ethnicities before approval. Meanwhile, the EU’s AI Act, set for 2025 implementation, classifies medical AI as “high risk,” mandating rigorous audits and transparency reports.
Industry responses vary. Some firms, like Germany’s Ada Health, welcome stricter rules. Their symptom-checker app, used by 12 million patients, now publishes bias mitigation strategies quarterly. Others resist. A 2023 survey by PwC found 34% of pharma executives view compliance costs as prohibitive, risking innovation slowdowns. Balancing safety and progress remains contentious.
Case Study: AI in Rare Pediatric Diseases
Few areas need AI more urgently than rare pediatric conditions. With 95% lacking treatments, families often face diagnostic odysseys lasting years. Paris-based startup Owkin tackles this via its AI platform, partnering with 300 hospitals worldwide. In 2022, the firm identified a gene variant linked to a fatal childhood muscle disorder, leading to a gene therapy trial funded by France’s National Research Agency.
Another pioneer, Italy’s Mendelian, uses NLP to mine clinical notes for undiagnosed cases. Their system flagged 12 children in Spain with a rare metabolic disorder in 2023, enabling early intervention. “Speed is everything,” says CEO Ignacio Hernández Medrano. “For these kids, a two-year delay can be fatal.”
Image Credit - BBC
Investment Surges and Economic Implications
Venture capital floods AI healthtech, with funding hitting £12.6 billion globally in 2023—a 46% annual rise. SoftBank’s Vision Fund alone injected £800 million into AI drug discovery startups, including Israel’s CytoReason, which models immune system interactions. Public markets follow suit. Nasdaq’s AI Healthcare Index surged 67% in 2023, outperforming the broader market by 41 points.
Critics warn of a bubble. A 2023 Goldman Sachs report noted that 60% of AI health startups lack revenue, relying on speculative patents. Still, governments bet big. In 2022, the UK allocated £250 million to its AI in Health and Care Awards, backing projects like Kheiron Medical’s cancer screening tools.
The Human Factor: Augmenting, Not Replacing, Clinicians
Amid automation fears, experts stress AI’s role as a collaborator. “Machines handle data; humans handle nuance,” says Dr. Sailem of King’s College. At Boston’s Brigham and Women’s Hospital, radiologists using AI detect 20% more early-stage lung cancers but still override 15% of AI suggestions. “The algorithm misses contextual clues—a patient’s smoking history, for instance,” explains lead radiologist Dr. Giles Stevenson.
Training evolves too. Edinburgh University’s medical school integrates AI modules, teaching students to interpret algorithm outputs. “Future doctors must be tech-savvy guides,” says Dean Dr. Moira Whyte. A 2023 survey of NHS staff found 68% welcome AI assistance but demand training programs—a need the UK government pledged £120 million to address last year.
AI in Real-Time Surgical Decision-Making
Surgeons removing brain tumours face a critical challenge: distinguishing cancerous cells from healthy tissue during operations. In 2024, an AI system called FastGlioma emerged as a game-changer. Developed by researchers at the University of Michigan and University of California, San Francisco, this tool analyses microscopic images of tissue samples in under 10 seconds. During simulated trials involving 624 specimens, FastGlioma achieved 98% accuracy in detecting residual cancer cells, outperforming traditional methods by 26 percentage points. The system, trained on 11,000 surgical samples, now guides neurosurgeons in 15 hospitals worldwide, reducing repeat surgeries by 40% in early adoption sites.
This innovation mirrors broader trends. For instance, London’s Kheiron Medical uses AI to review mammograms, flagging 12% more early-stage breast cancers than human radiologists in a 2023 NHS pilot. Such tools don’t replace clinicians but sharpen their precision. As Dr. Shaun Hervey-Jumper, a neurosurgeon involved in FastGlioma’s development, notes: “AI acts as a second pair of eyes, catching what we might miss under time pressure.”
Advancements in Predictive Oncology
Single-cell RNA sequencing has unlocked new frontiers in cancer treatment. In May 2024, the NIH-backed PERCEPTION tool demonstrated how AI could predict drug responses at the individual cell level. By analysing gene activity in 44 FDA-approved cancer drugs, the model achieved an 80% accuracy rate in forecasting which therapies would shrink tumours. One trial involving 41 multiple myeloma patients showed PERCEPTION correctly identified effective drug combinations in 85% of cases, slashing average trial-and-error periods from six months to three weeks.
Meanwhile, startups like Owkin leverage federated learning—where algorithms train on decentralised data—to study rare cancers. Their 2023 collaboration with 300 European hospitals accelerated the discovery of a gene therapy for paediatric muscle disorders, moving from lab to human trials in 11 months. “AI lets us bypass decades of guesswork,” says Dr. Eytan Ruppin, a lead researcher at the National Cancer Institute.
Democratising AI in Global Health
While wealthy nations pioneer AI healthcare, emerging economies adapt these tools to local needs. Rwanda’s 2023 partnership with Google Health tracks malaria outbreaks via smartphone data, directing antimalarial drugs to hotspots with 92% efficiency. Similarly, India’s 2024 national AI health initiative allocates £1.1 billion to develop low-cost diagnostic tools, aiming to cut drug import reliance by 30% by 2030.
Yet disparities persist. A 2024 WHO report found that 78% of AI medical research focuses on diseases prevalent in high-income countries. Initiatives like the EU’s Global Health Data Network aim to rebalance this, pooling anonymised data from 50 nations to train inclusive algorithms. Genetika+’s pilot in Nairobi clinics, launching in late 2024, tests AI-driven depression treatments tailored to genetic profiles underrepresented in Western datasets.
The Road to Ethical AI Adoption
Regulators race to ensure AI’s safety without stifling innovation. The EU’s AI Act, effective from 2025, mandates strict audits for medical algorithms, requiring developers to disclose training data sources and error rates. In the UK, the MHRA’s 2023 guidelines demand real-world performance reports every six months for approved AI tools. Such measures respond to incidents like IBM Watson’s 2021 recall of an oncology algorithm that misdiagnosed 15% of lung cancer cases due to biased training data.
Transparency remains pivotal. Insilico Medicine now publishes “AI bias scorecards” for its drug candidates, detailing how models address racial and gender disparities. “Trust hinges on accountability,” asserts Dr. Heba Sailem. “Patients deserve to know why an AI recommends one treatment over another.”
Conclusion: Balancing Innovation and Caution
Artificial intelligence undeniably reshapes how drugs are matched to patients, offering hope where traditional methods falter. From slashing clinical trial costs to personalising cancer therapies, the technology’s potential seems boundless. Yet as Calum Chace warns, “AI is a tool, not a panacea.” Success hinges on addressing ethical pitfalls—data biases, regulatory gaps, and access inequities—while preserving human oversight.
The next decade will test whether global healthcare systems can harness AI responsibly. Initiatives like the EU’s Health Data Space and Rwanda’s malaria projects show collaborative paths forward. For pioneers like Dr. Talia Cohen Solal, the goal remains clear: “AI should empower clinicians, not replace them. When used wisely, it bridges the gap between lab breakthroughs and real-world healing.” As trials evolve and algorithms refine, this balance between silicon and synapse may well define medicine’s future.
Recently Added
Categories
- Arts And Humanities
- Blog
- Business And Management
- Criminology
- Education
- Environment And Conservation
- Farming And Animal Care
- Geopolitics
- Lifestyle And Beauty
- Medicine And Science
- Mental Health
- Nutrition And Diet
- Religion And Spirituality
- Social Care And Health
- Sport And Fitness
- Technology
- Uncategorized
- Videos