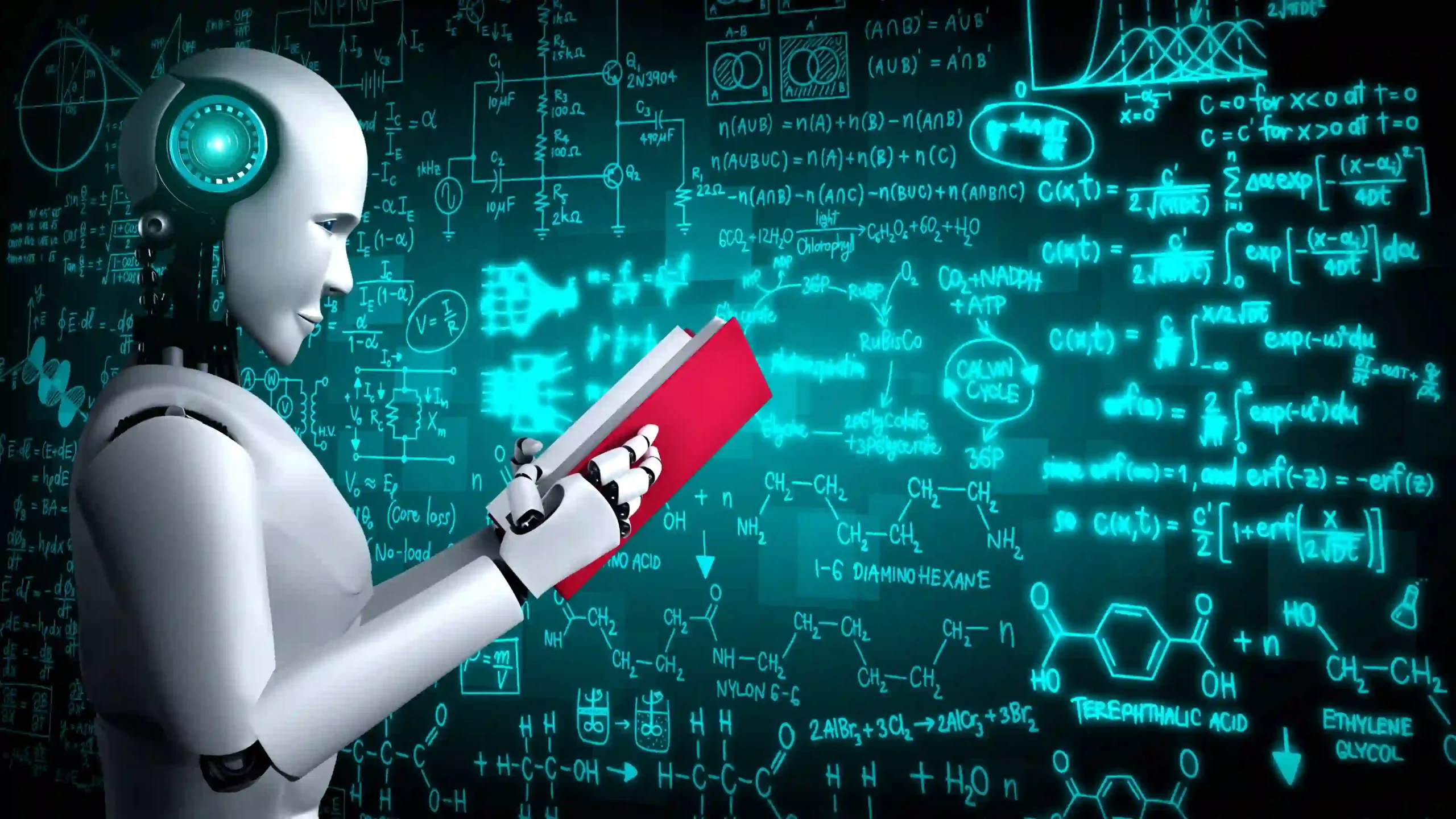
Limitations of AI in Mathematical Problem Solving
A.I.'s Mathematical Shortcomings: A Challenge and an Opportunity
In the realm of artificial intelligence (A.I.), a curious paradox has emerged. While these digital minds have demonstrated remarkable prowess in language-based tasks, crafting eloquent prose and engaging in meaningful conversations, they often stumble when confronted with the seemingly straightforward world of mathematics. This mathematical Achilles' heel, while puzzling, offers valuable insights into the nature of A.I. and its potential impact on fields ranging from education to scientific research.
Unlike humans, who can intuitively grasp mathematical concepts and apply them to various problems, A.I. systems, particularly those based on large language models, lack this innate understanding. They are trained on vast datasets of text and code, allowing them to predict patterns and generate human-like responses. However, this reliance on statistical probability rather than logical reasoning proves to be a hindrance when it comes to the precision and rigour required in mathematical calculations.
The Language-Math Divide: A Fundamental Difference
The disparity in A.I.'s performance between linguistic and mathematical tasks can be attributed to the fundamental differences between these two domains. Language, while complex, is inherently flexible and allows for a degree of ambiguity and interpretation. In contrast, mathematics is a domain of absolute truths, where even the slightest error can lead to wildly incorrect results. This makes it a challenging area for A.I. systems that are primarily designed to excel in the realm of language.
Moreover, mathematical problems often require a multi-step approach, involving the application of logical reasoning and the ability to manipulate abstract symbols. While A.I. systems can be trained on vast datasets of mathematical problems, they often struggle when faced with novel or complex problems that require a deeper understanding of underlying principles. This limitation has been highlighted in recent studies, where A.I. chatbots have been shown to falter when asked to solve even simple arithmetic problems or word problems that require multiple steps to reach a solution.
The Impact on Education and Beyond
The A.I.'s mathematical shortcomings have significant implications for education, where these tools are increasingly being used to support student learning. While A.I. chatbots can provide valuable assistance in language-based subjects like literature or history, their limitations in mathematics pose a challenge for educators who rely on them for tutoring or assessment purposes. This has led some educational institutions to adopt a hybrid approach, using A.I. for language-based tasks and relying on traditional calculators or human tutors for mathematical support.
Beyond education, the A.I.'s mathematical limitations also raise concerns about their potential impact on fields that heavily rely on mathematical models and calculations, such as finance, engineering, and scientific research. While A.I. has shown promise in areas like data analysis and pattern recognition, its inability to reliably perform complex mathematical operations raises questions about its ability to fully replace human experts in these fields.
In conclusion, the A.I.'s mathematical struggles, while a current limitation, also present an opportunity for further research and development. By understanding the underlying reasons for this disparity, researchers can develop new approaches to enhance A.I.'s mathematical capabilities, potentially opening up new avenues for its application in various fields. As A.I. continues to evolve, it is crucial to recognize both its strengths and weaknesses, ensuring that we utilize its capabilities in a responsible and effective manner.
Image Credit - Freepik
The Quest for Mathematical A.I.: Navigating the Challenges
Despite the current limitations, the quest for A.I. systems that can truly excel at mathematics is far from over. Researchers are actively exploring various approaches to bridge the gap between language and mathematical reasoning. One promising avenue is the development of hybrid systems that combine the strengths of large language models with more traditional, rule-based algorithms. These hybrid models could leverage the language model's ability to understand and interpret mathematical problems while relying on the algorithmic component for precise calculations.
Another approach involves incorporating external tools and resources into A.I. systems. This could include access to calculators, mathematical libraries, or even human experts who can provide guidance and feedback. Such an approach would allow A.I. systems to leverage the vast knowledge and computational power available in the digital world while mitigating their own limitations in mathematical reasoning.
Furthermore, researchers are also exploring the use of reinforcement learning techniques to train A.I. systems to solve mathematical problems. By providing feedback and rewards for correct answers, these systems can gradually learn to improve their performance over time. This approach has shown promising results in other domains, such as game playing, and could potentially be adapted to the realm of mathematics.
The Role of Data and Algorithms: A Delicate Balance
The success of A.I. in mathematics also hinges on the availability of high-quality data and the development of effective algorithms. Large language models require vast amounts of training data to learn patterns and make accurate predictions. In the case of mathematics, this data could include textbooks, research papers, and even online forums where mathematical problems are discussed and solved. By analyzing this data, A.I. systems can gain a deeper understanding of mathematical concepts and their applications.
However, data alone is not enough. Effective algorithms are also crucial for translating this data into meaningful insights and solutions. These algorithms need to be designed to handle the complexities and nuances of mathematical reasoning, taking into account the various types of problems, the different levels of difficulty, and the specific requirements of each domain.
The Future of Mathematical A.I.: A Collaborative Effort
The development of A.I. systems that can truly excel at mathematics is a complex and multifaceted challenge. It requires a collaborative effort between researchers, educators, and industry experts, all working together to overcome the current limitations and unlock the full potential of this technology. By combining the strengths of different approaches, leveraging the power of data and algorithms, and fostering collaboration across disciplines, we can pave the way for a future where A.I. not only understands language but also masters the language of mathematics.
Image Credit - Freepik
Overcoming Bias and Ensuring Fairness: A Critical Concern
As A.I. systems become more integrated into our lives, it is crucial to address the potential biases that can arise in their mathematical reasoning. These biases can stem from the data used to train the models, the algorithms themselves, or even the human designers who create them. For instance, if an A.I. system is trained on data that is skewed towards certain demographics or socioeconomic groups, it may inadvertently perpetuate these biases in its mathematical calculations and predictions.
To ensure fairness and equity, researchers are actively working on developing methods to identify and mitigate biases in A.I. systems. This involves carefully scrutinizing the data used for training, ensuring diversity and representation, and regularly evaluating the system's performance across different groups. Additionally, incorporating transparency and explainability into A.I. algorithms can help users understand the reasoning behind the system's mathematical calculations, making it easier to identify and correct any potential biases.
A.I. in Mathematics Education: Transforming the Learning Experience
While the A.I.'s mathematical shortcomings pose a challenge for education, they also present an opportunity to reimagine how mathematics is taught and learned. By leveraging the strengths of A.I., educators can create personalized learning experiences that cater to the individual needs and strengths of each student. For example, A.I. tutors can provide targeted feedback and support, helping students overcome their specific challenges and build a solid foundation in mathematics.
Furthermore, A.I. can be used to create engaging and interactive learning environments, where students can explore mathematical concepts through simulations, games, and other interactive activities. This can make mathematics more accessible and enjoyable for students, fostering a deeper understanding and appreciation for the subject.
The Role of Human Expertise: A Valuable Asset
Despite the advances in A.I., human expertise remains a valuable asset in the realm of mathematics. While A.I. systems can perform complex calculations and analyze vast amounts of data, they still lack the intuition, creativity, and critical thinking skills that are essential for solving novel and complex mathematical problems.
Therefore, it is crucial to foster collaboration between A.I. systems and human experts, leveraging the strengths of each to achieve optimal results. For instance, A.I. systems can be used to automate routine tasks, freeing up human experts to focus on more complex and creative endeavors. Additionally, A.I. can provide valuable insights and recommendations, helping human experts make informed decisions and solve challenging problems.
Ethical Considerations and Responsible A.I. Development
As A.I. systems continue to advance in their mathematical capabilities, it is imperative to address the ethical considerations associated with their development and deployment. One key concern is the potential for A.I. to exacerbate existing inequalities in access to education and resources. If A.I.-powered educational tools are not accessible to all students, it could further widen the achievement gap between those who have access to these resources and those who do not.
To ensure equitable access, it is crucial to develop A.I. systems that are affordable, user-friendly, and adaptable to different learning environments. This may involve creating open-source platforms, providing training and support for educators, and partnering with organizations that can help distribute these tools to underserved communities.
Another ethical consideration is the potential for A.I. to be used for malicious purposes, such as cheating on exams or manipulating financial data. To mitigate these risks, it is important to develop robust security measures and ethical guidelines for the use of A.I. in mathematics. This could involve implementing authentication protocols, watermarking A.I.-generated content, and educating users about the potential risks and limitations of these tools.
Image Credit - Freepik
Limitations of AI in Expanding the Future of Mathematical Research
The integration of A.I. into mathematical research has the potential to revolutionize the field, opening up new avenues for discovery and innovation. By automating routine tasks, A.I. can free up mathematicians to focus on more complex and creative problems. For example, A.I. systems can be used to verify proofs, generate conjectures, and even discover new mathematical theorems.
Furthermore, A.I. can help bridge the gap between different branches of mathematics, identifying connections and patterns that may not be immediately apparent to human researchers. By analyzing vast amounts of mathematical data, A.I. systems can uncover hidden relationships and generate new insights, leading to breakthroughs in fields as diverse as number theory, topology, and algebraic geometry.
Limitations and the Symbiotic Relationship Between Humans and AI
While A.I. has the potential to transform mathematics, it is important to remember that it is not a replacement for human ingenuity and creativity. Instead, A.I. should be seen as a powerful tool that can augment and enhance human capabilities, enabling us to tackle problems that were previously intractable.
By working together, humans and A.I. can achieve a symbiotic relationship, where each complements the other's strengths and weaknesses. Humans can provide the intuition, creativity, and critical thinking skills that are essential for mathematical discovery, while A.I. can provide the computational power, data analysis capabilities, and pattern recognition abilities that can accelerate and enhance this process.
Image Credit - Freepik
A.I. in the Real World: Applications and Limitations
The practical applications of A.I. in mathematics are already being realized in various fields. In finance, A.I.-powered algorithms are used for risk assessment, portfolio optimization, and fraud detection. In engineering, A.I. is used to design complex structures, optimize manufacturing processes, and predict the behavior of materials under different conditions. In scientific research, A.I. is used to analyze large datasets, model complex phenomena, and even discover new drugs and materials.
However, despite these successes, the limitations of A.I. in mathematics are still evident. In fields like pure mathematics, where abstract concepts and rigorous proofs are paramount, A.I. is still far from replacing human mathematicians. The creative spark, intuitive leaps, and deep understanding that characterize human mathematical thinking remain elusive for A.I. systems.
Moreover, the reliance of A.I. on data and algorithms raises concerns about the potential for errors and biases. In real-world applications, where the stakes are high, even minor errors in mathematical calculations can have significant consequences. Therefore, it is crucial to develop robust validation and verification mechanisms to ensure the accuracy and reliability of A.I.-powered mathematical tools.
Conclusion: The Path Forward
The A.I.'s journey in the realm of mathematics is a testament to the rapid advancements in this field. While the current limitations in mathematical reasoning pose a challenge, they also present an opportunity for further research and development. By exploring new approaches, leveraging the power of data and algorithms, and fostering collaboration between humans and A.I., we can unlock the full potential of this technology and transform the way we approach mathematics.
The future of A.I. in mathematics is bright, with endless possibilities for innovation and discovery. As we continue to push the boundaries of what A.I. can achieve, it is crucial to prioritize ethical considerations, ensure fairness and equity, and foster a collaborative spirit between humans and machines. By doing so, we can create a future where A.I. not only augments our mathematical abilities but also inspires us to think in new and creative ways, leading to a deeper understanding and appreciation for the beauty and power of mathematics.
Recently Added
Categories
- Arts And Humanities
- Blog
- Business And Management
- Criminology
- Education
- Environment And Conservation
- Farming And Animal Care
- Geopolitics
- Lifestyle And Beauty
- Medicine And Science
- Mental Health
- Nutrition And Diet
- Religion And Spirituality
- Social Care And Health
- Sport And Fitness
- Technology
- Uncategorized
- Videos