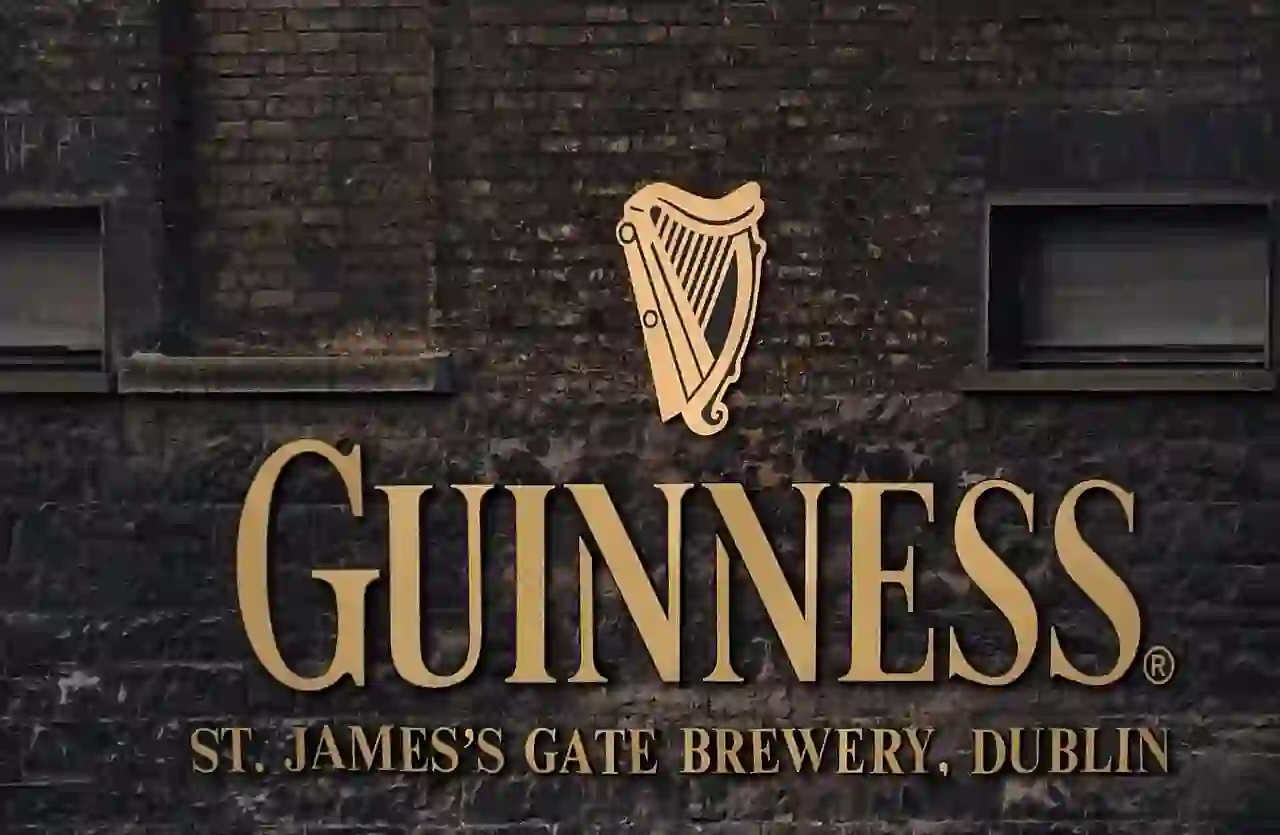
Guinness Brewery A Legacy in Statistical Significance
The Guinness Brewery: A Hub of Innovation Beyond Brewing
The Guinness Brewery, renowned for its iconic stout and 9,000-year lease on a Dublin site, is more than a purveyor of fine ales. Indeed, it's a hotbed of innovation that has significantly impacted the world beyond brewing. While the brewery's founder, Arthur Guinness, may have been focused on crafting the perfect pint, the company he established has fostered a legacy of scientific discovery.
The Quest for the Perfect Pint: A Catalyst for Scientific Discovery
In the early 20th century, Guinness, already the world's largest brewery, embarked on a quest for unparalleled quality control. This ambition led to the assembly of a team of brilliant minds, tasked with applying scientific rigor to the art of brewing. Their mission was to answer questions that ranged from the ideal barley varieties to the impact of advertising campaigns on sales.
The Challenge of Small Sample Sizes: A Statistical Quandary
In their pursuit of brewing perfection, the Guinness scientists encountered a recurring challenge: how to interpret data from small sample sizes. For instance, when assessing the quality of hops, a key ingredient in Guinness, brewers measured the soft resin content in a limited number of plants. If the average resin content in these samples deviated significantly from the desired level, should they discard the entire crop? Or could the low readings simply be due to chance, given the small sample size?
The T-Test: A Statistical Breakthrough Born in a Brewery
This statistical conundrum, pervasive in scientific research, led William Sealy Gosset, head experimental brewer at Guinness, to develop the t-test. This groundbreaking statistical method allowed scientists to determine whether observed differences between samples were statistically significant or merely due to random variation.
Understanding Statistical Significance: Signal vs. Noise
The concept of statistical significance revolves around the idea of a P-value, which represents the probability of observing a particular result if there's no real effect. Typically, a P-value below 0.05 is considered statistically significant. This threshold, however, can vary depending on the specific application.
The Role of Sample Size and Variation in Statistical Significance
Two factors influence the P-value: the magnitude of the difference between the observed and expected values (the signal) and the variability within the data (the noise). A larger difference between the observed and expected values suggests a real effect, while high variability within the data could mean the observed difference is due to chance.
The Normal Distribution and the T-Distribution: Tools for Assessing Significance
To determine statistical significance, scientists often use the normal distribution, a bell-shaped curve that describes the probability of different outcomes. However, Gosset realized that this approach was unsuitable for small sample sizes. He meticulously calculated new distributions, known as t-distributions, which are similar to the normal distribution but with wider tails. These t-distributions require a larger difference between the observed and expected values to reach statistical significance.
Gosset's Secret Weapon: The Pseudonym "Student"
Gosset's t-test revolutionised statistical analysis, particularly in situations with limited data. However, Guinness, not wanting to divulge its competitive advantage, insisted that Gosset publish his findings under the pseudonym "Student." This cloak of anonymity led to the technique being widely known as the "Student's t-test," obscuring Gosset's pivotal role in its development.
From Brewery to Broader Applications: The T-Test's Enduring Legacy
Despite the initial secrecy surrounding its origins, the t-test quickly gained recognition in the scientific community. Its versatility and applicability to a wide range of fields, from medicine to agriculture, cemented its status as an indispensable statistical tool. Today, the t-test is routinely used to assess the significance of experimental results, clinical trial outcomes, and survey data, among other applications.
Image Credit - Wikipedia
The T-Test in Action: Real-World Examples
To illustrate the t-test's practical significance, consider a medical trial comparing the effectiveness of a new drug to a standard treatment. By analysing the data from the trial using a t-test, researchers can determine whether the observed difference in outcomes between the two groups is statistically significant, indicating that the new drug is genuinely more effective.
The T-Test and Quality Control: Ensuring Consistency in Guinness
At Guinness, the t-test became an integral part of the brewery's quality control process. By analysing samples of hops, barley, and other ingredients, brewers could ensure that the raw materials met the brewery's stringent standards, contributing to the consistent quality of Guinness stout.
Beyond Brewing: The T-Test's Impact on Scientific Research
The t-test's impact extends far beyond the brewery. In agricultural research, for example, the t-test is used to compare the yields of different crop varieties, helping farmers select the most productive options. In environmental studies, the t-test can be used to assess the impact of pollution on ecosystems, providing valuable insights for conservation efforts.
The T-Test in the Digital Age: Statistical Analysis Made Easy
With the advent of powerful statistical software, conducting a t-test has become more accessible than ever before. Researchers can now quickly and easily analyse their data, regardless of their statistical expertise. This democratisation of statistical analysis has further expanded the reach and impact of the t-test.
The T-Test's Limitations: A Note of Caution
While the t-test is a valuable statistical tool, it's important to acknowledge its limitations. The t-test assumes that the data are normally distributed, which may not always be the case. Additionally, the t-test is sensitive to outliers, which can skew the results. Therefore, it's crucial to carefully consider the assumptions of the t-test and the characteristics of the data before applying it.
Misinterpretations and Misuses: The T-Test's Dark Side
Despite its widespread use and undeniable value, the t-test is not immune to misinterpretations and misuses. One common misconception is that a statistically significant result automatically implies a large or meaningful effect. However, statistical significance merely indicates that an observed difference is unlikely due to chance, not necessarily that it is practically important.
Moreover, the t-test is often misused as a fishing expedition, where researchers repeatedly test different hypotheses until they find a statistically significant result. This practice, known as p-hacking, can lead to false positives and misleading conclusions. It's essential to remember that the t-test is a tool for hypothesis testing, not hypothesis generation.
Beyond the T-Test: The Evolution of Statistical Methods
While the t-test remains a cornerstone of statistical analysis, it's important to recognize that it's just one tool in a vast toolbox. Over the years, statisticians have developed a wide array of methods to address different types of data and research questions. These methods include ANOVA, chi-squared tests, regression analysis, and many others.
Each statistical method has its own strengths and limitations, and choosing the right one depends on the specific research question, the type of data, and the underlying assumptions. As statistical software continues to evolve, researchers have access to increasingly sophisticated tools that can help them make sense of complex data and draw meaningful conclusions.
The Future of Statistical Analysis: Embracing Complexity
The field of statistics is constantly evolving, with new methods and techniques emerging to address the challenges of modern research. As datasets become larger and more complex, statisticians are developing innovative approaches to handle big data, high-dimensional data, and non-linear relationships.
Machine learning, a branch of artificial intelligence, is also playing an increasingly important role in statistical analysis. By leveraging algorithms and computational power, machine learning can uncover patterns and relationships in data that would be impossible to detect using traditional statistical methods.
The Guinness Legacy: A Toast to Innovation
The story of the t-test is a testament to the power of curiosity, ingenuity, and the unexpected connections between seemingly disparate fields. From the humble beginnings of a brewery's quest for quality control, the t-test has emerged as a cornerstone of scientific research, enabling discoveries that have transformed our understanding of the world.
As we raise a glass to William Sealy Gosset and the Guinness brewery, let us celebrate the spirit of innovation that continues to drive scientific progress. The t-test, born in a brewery and nurtured by a thirst for knowledge, is a shining example of how seemingly simple ideas can have a profound and lasting impact on our world.
The T-Test in the Age of Big Data: A Statistical Workhorse
In today's data-driven world, the t-test continues to be a statistical workhorse, albeit with some caveats. With the advent of big data, where datasets can contain millions or even billions of observations, the t-test's reliance on small sample assumptions can be a limiting factor.
In such scenarios, alternative statistical methods, such as the z-test, which is designed for large sample sizes, may be more appropriate. Additionally, advanced statistical techniques like bootstrapping and permutation tests offer robust alternatives to the t-test when dealing with non-normal distributions or complex data structures.
The T-Test and the Replication Crisis: A Call for Rigor
The t-test has also been implicated in the ongoing replication crisis in science, where many published findings fail to replicate in subsequent studies. One contributing factor is the misuse of the t-test and the overreliance on statistical significance as the sole criterion for scientific validity.
To address this issue, there is a growing movement towards greater transparency and rigor in statistical analysis. This includes pre-registering research hypotheses, making data and analysis code publicly available, and adopting more stringent statistical thresholds for significance.
Image Credit - Diageo Bar Academy
The T-Test in the Classroom: A Foundation for Statistical Literacy
Despite its limitations and potential for misuse, the t-test remains a fundamental concept in statistics education. By teaching students about the t-test, educators can introduce them to the principles of hypothesis testing, statistical inference, and the importance of critical thinking in data analysis.
Moreover, the story of the t-test's origin in a brewery can serve as an engaging and relatable example to spark students' interest in statistics. By highlighting the real-world applications of statistical methods, educators can make statistics more accessible and relevant to students from diverse backgrounds.
The T-Test's Enduring Appeal: Simplicity and Versatility
One of the reasons for the t-test's enduring popularity is its relative simplicity and ease of use. Compared to more complex statistical methods, the t-test is relatively straightforward to understand and apply, making it accessible to researchers and practitioners across various disciplines.
Furthermore, the t-test's versatility allows it to be applied to a wide range of research questions and data types. Whether you're comparing the effectiveness of two treatments, assessing the impact of a policy intervention, or evaluating the results of a survey, the t-test can provide valuable insights into the significance of your findings.
Image Credit - Wikipedia
The T-Test in the Era of Open Science: A Tool for Collaboration
In the era of open science, where data sharing and collaboration are increasingly encouraged, the t-test has found new relevance. By making data and analysis code publicly available, researchers can enable others to verify their findings, replicate their studies, and build upon their work.
This transparency not only enhances the credibility of scientific research but also fosters a culture of collaboration and knowledge sharing. The t-test, as a widely understood and accessible statistical method, plays a crucial role in facilitating this open exchange of scientific information.
The T-Test and the Democratization of Data Analysis
The rise of user-friendly statistical software and online resources has made the t-test and other statistical methods more accessible to a wider audience. Non-statisticians, such as researchers in other fields, students, and even citizen scientists, can now leverage the power of statistical analysis to gain insights from their data.
This democratization of data analysis has the potential to empower individuals and communities to make informed decisions based on evidence, rather than relying on intuition or anecdotal information. The t-test, with its intuitive appeal and ease of use, is a valuable tool in this ongoing process of empowering people with data.
Image Credit - Wikipedia
The T-Test: A Bridge Between Theory and Practice
The t-test is not just a theoretical construct; it's a practical tool that has real-world implications. From guiding medical treatments to informing policy decisions, the t-test helps us make sense of the world around us and make informed choices based on evidence.
By bridging the gap between theory and practice, the t-test empowers us to apply statistical principles to real-world problems, making a tangible difference in people's lives.
Conclusion: The T-Test's Timeless Legacy
The t-test, born in a brewery over a century ago, continues to be an essential tool in the arsenal of modern science. Its versatility, simplicity, and applicability to a wide range of research questions have made it a staple in fields as diverse as medicine, agriculture, economics, and psychology.
While the t-test is not without its limitations and potential for misuse, its enduring legacy is a testament to its fundamental importance in statistical analysis. As we continue to grapple with the challenges of big data, replication crises, and the need for greater transparency and rigor in research, the t-test will undoubtedly continue to play a crucial role in advancing our understanding of the world.
In the end, the t-test is more than just a statistical method; it's a symbol of human ingenuity, the power of collaboration, and the enduring quest for knowledge. From its humble origins in a Dublin brewery to its widespread use in scientific research and beyond, the t-test stands as a testament to the enduring impact of simple ideas and the power of statistics to illuminate the complexities of our world.
Recently Added
Categories
- Arts And Humanities
- Blog
- Business And Management
- Criminology
- Education
- Environment And Conservation
- Farming And Animal Care
- Geopolitics
- Lifestyle And Beauty
- Medicine And Science
- Mental Health
- Nutrition And Diet
- Religion And Spirituality
- Social Care And Health
- Sport And Fitness
- Technology
- Uncategorized
- Videos